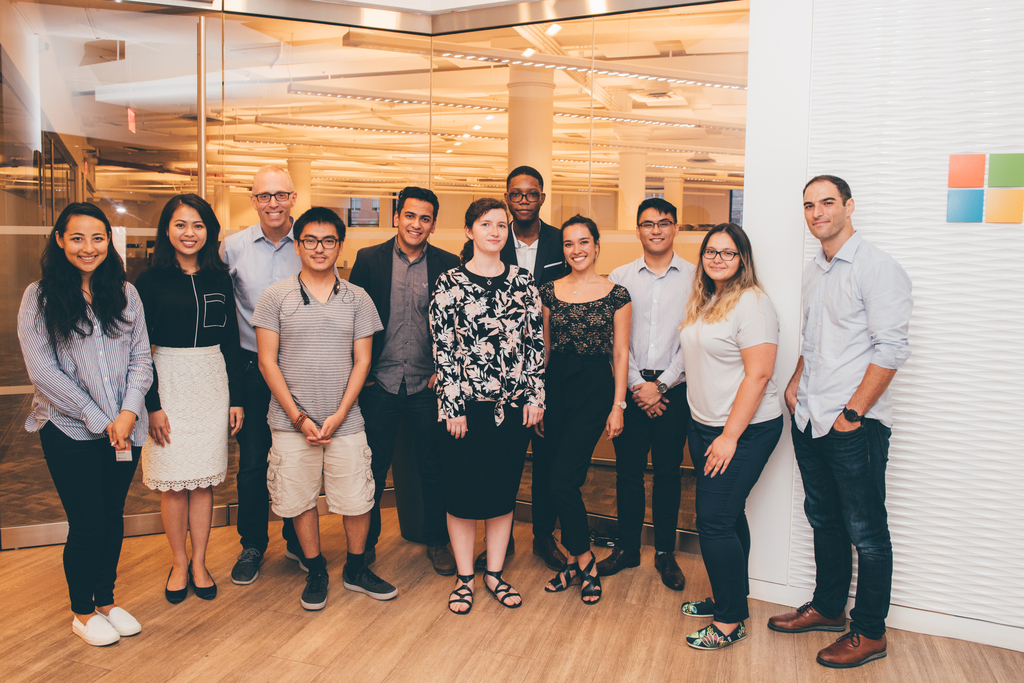
DS3 2018 at Microsoft Research New York City
This month marked the 5th anniversary of the Microsoft Research Data Science Summer School (DS3). DS3 is an intensive, eight-week hands-on introduction to data science for college students in the New York City area committed to increasing diversity in computer science. The program is taught by leading scientists at Microsoft Research and is held at the Microsoft Research New York City lab.
Each year the program receives upwards of 200 applications, out of which only eight students, demonstrating academic excellence and a passion for using technology to help society, are selected to participate. These students complete four weeks of intensive course work and spend the remaining four weeks of their summer working on an original research problem. Graduates of the program have gone on to a number of exciting careers, ranging from data scientist positions at companies like Microsoft, Bloomberg, and American Express to PhD programs at universities such as Cornell and NYU.
Past projects have looked at how students progress through the New York City public school system, investigated demographic disparities in the city’s policing activities, and formulated improvements for the city’s taxi fleet and bike sharing service.
This year’s students used their newly acquired data science skills to examine another way of getting around New York City—the city’s subway system—and presented some impressive findings at the DS3 banquet to an overflowing room of select members of New York City’s tech community. They examined rider wait times and trip times, compared the subway to above ground travel, and investigated how changes to the system affect rider options.
Below is a summary of their presentation, which you can watch in full. The project is also available on GitHub.
Akbar Mirza, a senior from City College, opened the talk by discussing the history of NYC’s subway system, which is the largest rapid transit system in the world, serving approximately 5.5 million riders each day. He highlighted the growing concern that the system has become unreliable due to aging equipment, some of which dates back to the early 20th century. And while current system-wide metrics provide some insight into the state of the subway system, they fail to capture how riders actually experience the subway.

This motivated the students to investigate the subway system using the data behind the system’s new countdown clocks that record train locations. Specifically, they used a dataset collected and cleaned by local data scientist Todd Schneider that contained the approximate location of every train in the system for every minute of each day from January through May of 2018.
Next, Brian Hernandez, a senior from Hunter College, walked the audience through how this data could be used to understanding how long riders spend waiting for trains. He used these calculations to compare his commuting options on the F and 7 trains, showing that while the typical wait time is the same on both lines, the F train has much higher variability than the 7 train, making the 7 the preferred option.

Amanda Rodriguez, a senior at Lehman College, continued the presentation with a more granular look at subway wait times throughout the city. She presented a comprehensive wait time model that considers station- and line-specific factors as well as day of week, time of day, and weather effects. Her analysis revealed interesting patterns in wait time variability throughout the city and showed that heavy rain can result in as much as a 25% increase in typical wait times at certain locations.

Taxi Baerde, a senior from Adelphi University, introduced the next topic—constructing a formal representation of the subway network as a graph that could be used for finding shortest paths between any two stops and computing trip times. Taxi discussed how it’s surprisingly difficult to settle on such a representation because the network itself is so dynamic, with changing schedules, partial routes, and skipped stops. He also presented a method, called k-shortest paths, for identifying different possible itineraries between a pair of stations (for instance, taking the local versus express, or transferring between multiple likes).

Next, Phoebe Nguyen, a junior at Baruch College, showed how Taxi’s cleaned subway graph could be used to compare different commuting options between a pair of stations in a two- step process—first, finding a set of candidate paths between the stations; and second, reconstructing how long it actually took trains to make these trips. She used this method to compare different options for various trips, showing once again that variability is often the key for deciding between two different options.

Peter Farquharson, a junior from Lehman College, extended Phoebe’s results to answer a question on many busy New Yorkers’ minds: when is the subway a better option than a car? He demonstrated how open data from the city’s Taxi and Limosine Commission could be used to estimate how long past car trips between two subway stations would have taken, and compared this with corresponding subway trips. His results highlighted that, once variability is factored in, the subway can be an attractive alternative to driving when trying to get to midtown Manhattan during rush hour or traveling to JFK airport.

Ayliana Teitelbaum, a sophomore from Yeshiva University, looked at trip times from a different angle to tackle a question that New Yorkers face in choosing where to live—how long should you expect your commute to take coming from different parts of the city? She extended Phoebe’s results by showing historical trip times between each of the nearly 500 stations in the system to a fixed workplace destination, and presented the results as a heatmap. By comparing typical and worst case commute times for each station, she showed that accounting for variability can increase commute times in the outer boroughs by up to 50%.

Sasha Paulovich, a senior at Fordham University, presented the final set of results, considering how changes to the subway system affect riders and how subway experiences differ across demographic groups. She presented a heatmap similar to Ayliana’s that showed how we can expect commute times to change after the L train shuts down in January 2019, and an analogous map that projected commute times to LaGuardia airport if the proposed AirTrain extension to Willets Point is built. Finally, she discussed station options and commute times for riders requiring accessible stations and showed a correlation between median household income and commute times.

The team and their Microsoft Research mentors closed out the evening by fielding a host of questions from the audience, where the students discussed all of the additional topics they thought about tackling and the various extensions and future work to be done.
This content was originally published here.